AI companies hit a scaling wall
OpenAI, Google and others are seeing diminishing returns to building ever-bigger models — but that may not matter as much as you would guess
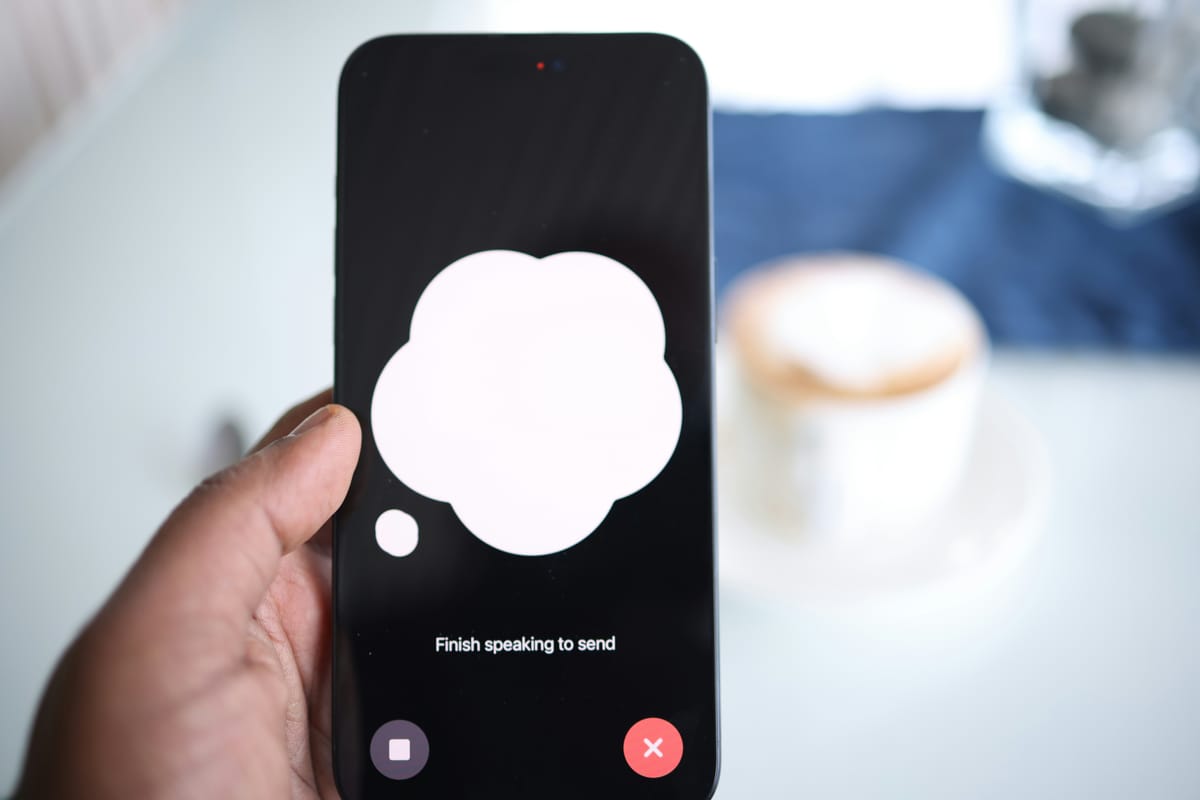
I.
Over the past week, several stories sourced to people inside the big AI labs have reported that the race to build superintelligence is hitting a wall. Specifically, they say, the approach that has carried the industry from OpenAI’s first large language model to the LLMs we have today has begun to show diminishing returns.
Today, let’s look at what everyone involved is saying — and consider what it means for the AI arms race. While reports that AI scaling laws appear to be technically accurate, they can also be easily misread. For better and for worse, it seems, the development of more powerful AI systems continues to accelerate.
Scaling laws, of course, are not laws in the sense of “laws of nature.” Rather, like Moore’s law, they contain an observation and a prediction. The observation is that LLMs improve as you increase the size of the model, the amount of data fed into the model for training and testing, and the computational resources needed to complete the training run.
First documented in a paper published by OpenAI in 2020, the laws have a powerful hold on the imagination of people who work on AI (and those who write about it). If the laws continue to hold true through the next few generations of ever-larger models, it seems plausible that one of the big AI companies might indeed create something like superintelligence. On the other hand, if they begin to break down, those same companies might face a much harder task. Scaling laws are fantastically expensive to pursue, but technically quite well understood. Should they falter, the winning player in the AI arms race may no longer be the company that spends the most money the fastest.
Last week, The Information reported that OpenAI may have begun to hit such a limit. Here are Stephanie Palazzolo, Erin Woo, and Amir Efrati:
In May, OpenAI CEO Sam Altman told staff he expected Orion, which the startup’s researchers were training, would likely be significantly better than the last flagship model, released a year earlier. [...]
While Orion’s performance ended up exceeding that of prior models, the increase in quality was far smaller compared with the jump between GPT-3 and GPT-4, the last two flagship models the company released, according to some OpenAI employees who have used or tested Orion.
Some researchers at the company believe Orion isn’t reliably better than its predecessor in handling certain tasks, according to the employees. Orion performs better at language tasks but may not outperform previous models at tasks such as coding, according to an OpenAI employee.
Reuters added fuel to the fire this week with a piece that quoted Ilya Sutskever, the OpenAI co-founder who left earlier this year to found his own AI lab, seeming to confirm the idea that scaling laws are hitting their limits. Here are Krystal Hu and Anna Tong:
Ilya Sutskever, co-founder of AI labs Safe Superintelligence (SSI) and OpenAI, told Reuters recently that results from scaling up pre-training — the phase of training an AI model that use a vast amount of unlabeled data to understand language patterns and structures — have plateaued. [...]
“The 2010s were the age of scaling, now we're back in the age of wonder and discovery once again. Everyone is looking for the next thing,” Sutskever said. “Scaling the right thing matters more now than ever.”
Finally, Bloomberg on Wednesday confirmed that OpenAI’s Orion had underperformed expectations and said Google and Anthropic had encountered similar difficulties. Here are Rachel Metz, Shirin Ghaffary, Dina Bass, and Julia Love:
At … Google, an upcoming iteration of its Gemini software is not living up to internal expectations, according to three people with knowledge of the matter. Anthropic, meanwhile, has seen the timetable slip for the release of its long-awaited Claude model called 3.5 Opus.
II.
So what’s happening? Experts offer several theories about why new models aren’t performing as well as their makers hope.
One is that AI companies are running out of high-quality new data sets to feed into their models. By this point, they’ve managed to scrape all of the low- and medium-hanging fruit on the web. Only recently did OpenAI, Meta and others begin paying to license high-quality data from publishers and other sources. Efforts to paper over this deficiency with synthetic data — data made by the AI labs themselves — have yet to generate a breakthrough.
Another, potentially more troublesome explanation is that superintelligence simply can’t be built with LLMs alone. Progress in AI is measured primarily in the ability of these models to hit various benchmarks. And while LLMs have been crushing benchmarks at impressive speed, the benchmarks can be gamed. More importantly, models fail to understand cause and effect and other tasks that require multi-step reasoning. They often make factual mistakes and lack real-world knowledge.
Perhaps the issue, as Tyler Cowen writes at Marginal Revolution today, is that we have taken too blinkered a view of “knowledge” — and placed impossible expectations on LLMs.
“Knowledge is about how different parts of a system fit together, rather than being a homegenous metric easily expressed on a linear scale,” he writes. “ There is no legible way to assess the smarts of any single unit in the system, taken on its own. Furthermore, there are many ‘walls,’ meaning knowledge is bumpy and lumpy under the best of circumstances. It thus makes little sense to assert that an entity is ‘3x smarter’ than before.”
If you are the sort of person who is concerned about AI’s rapid development, this could seem like welcome news. A roadblock on the way toward superintelligence means more time to bring systems into alignment with human values and prevent them from causing harm. It lets society adapt to the ways AI is already deeply affecting us, from education to publishing to politics. It lets us breathe.
But this may turn out to be a kind of wishful thinking.
III.
“There is no wall,” Sam Altman posted on X today.
It was the sort of gnomic pronouncement that Altman has increasingly favored this year, as he teases upcoming releases with photos of his garden and offers other Easter eggs toward his followers.
OpenAI had declined to comment on the reports above, but here was Altman appearing to deny them — or if not deny their individual claims, at least to deny their implications. OpenAI would not be hindered by any slowdown in the scaling laws, he seemed to say. And indeed, The Information reported that the company’s recent focus on its reasoning-focused o1 model represented one way the company is attempting to accelerate its progress again.
In its story, Reuters quoted Noam Brown, a researcher at OpenAI who worked on o1, at a TED AI conference last month in San Francisco. The o1 model uses a technique known as “test time compute,” which effectively gives the model more time and compute at the moment of inference. (In ChatGPT terms, inference is the moment when you hit enter on your query.)
According to Brown, the impact of test time compute can be extraordinary.
“It turned out that having a bot think for just 20 seconds in a hand of poker got the same boosting performance as scaling up the model by 100,000x and training it for 100,000 times longer,” he said at TED AI.
No wonder, then, that AI CEOs seem relatively unbothered by the scaling law slowdown. Anthropic CEO Dario Amodei appeared on Lex Fridman’s podcast this week, and offered a similarly upbeat take.
“We have nothing but inductive inference to tell us that the next two years are going to be like the last 10 years,” said Amodei, who co-authored that first paper on scaling laws while at OpenAI. “But I’ve seen the movie enough times, I’ve seen the story happen for enough times to really believe that probably the scaling is going to continue, and that there’s some magic to it that we haven’t really explained on a theoretical basis yet.”
There are, of course, self-interested reasons to promote the idea that the scaling laws are intact. (Anthropic is reportedly raising billions of dollars at the moment.) But Anthropic has not been prone to hyperbole, and takes AI safety more seriously than some of its peers. If AI progress were slowing, I suspect many people at the company might actually be relieved — and would say so.
Ultimately, we won’t be able to evaluate the next-generation models until the AI labs put them into our hands. On Thursday, an experimental new version of Google’s Gemini model surprised some by immediately vaulting to the top of a closely watched AI leaderboard, placing it ahead of ChatGPT 4o. In a post on X, CEO Sundar Pichai offered the up-and-to-the-right stock-chart emoji. “More to come,” he said.
Achieving superintelligence may indeed require breakthroughs that scaling laws alone cannot provide. But there may well be more than one path to the finish line. Which means, among other things, that we should not lose focus on what it would actually mean if we got there.
Elsewhere in AI progress:
- OpenAI is reportedly preparing to launch an AI agent, codenamed “Operator,” that can complete tasks like writing code and booking travel. (Shirin Ghaffary and Rachel Metz / Bloomberg)
- The ChatGPT desktop app for macOS can now read code for some coding apps on Macs, such as VS Code and Terminal. (Maxwell Zeff / TechCrunch)
- The Gemini AI app for iPhones is now available. (David Pierce / The Verge)
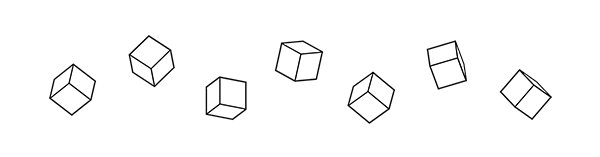
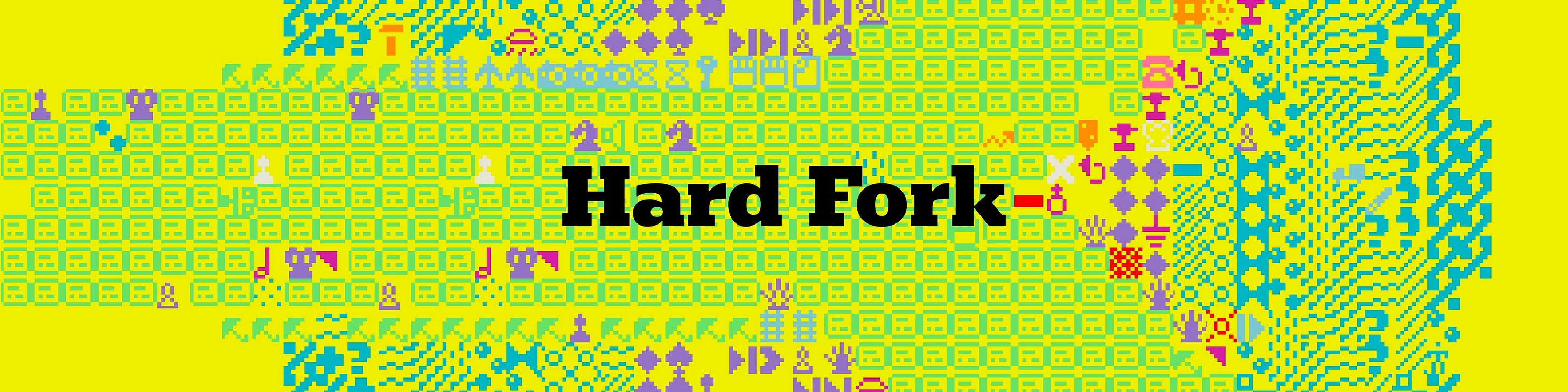
On the podcast this week: Kevin and I talk through the implications of the surprisingly pro-crypto Congress that will be seated in January. Then, ChatGPT product lead Nick Turley joins us to discuss the chatbot's first two years. And finally, we answer a listener's question about what social network they should be using today.
Apple | Spotify | Stitcher | Amazon | Google | YouTube
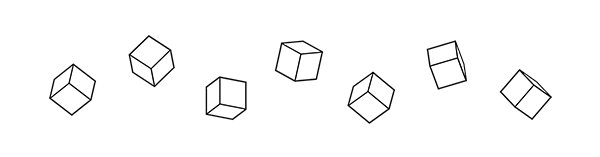
Governing
- A look at Elon Musk’s hands-on influence on the Trump transition effort and at Mar-a-Lago. It's ... quite extensive. (Theodore Schleifer / New York Times)
- A look at what experts think cybersecurity will be like in the new Trump term – relaxed rules around businesses and human rights, but a beefed-up front against foreign adversaries. (Eric Geller / Wired)
- The DOJ is reportedly investigating betting platform Polymarket on allegations that it accepted trades from US-based users, and has seized CEO Shayne Coplan’s phone and electronics. (Myles Miller and Lydia Beyoud / Bloomberg)
- X saw its largest decrease in users (since Musk bought it) on the day after the election, with many turning to Bluesky and Threads. (Kat Tenbarge and Kevin Collier / NBC News)
- The Guardian said it will no longer post on X over concerns about “disturbing content” and how Musk “has been able to use its influence to shape political discourse.” (The Guardian)
- Bluesky has added more than 1 million users to its platform in the week since the election, the company said. (Callie Holtermann / New York Times)
- Executives are reportedly planning to advertise on X again in an effort to curry favor with the new Trump administration. ?????? (Hannah Murphy, Daniel Thomas and Eric Platt / Financial Times)
- Satirical publication The Onion said it won a bankruptcy auction to buy Infowars, the site that conspiracy theorist Alex Jones has used to peddle disinformation about the Sandy Hook shooting. Best media story of the year, and it's not particularly close. (Benjamin Mullin and Elizabeth Williamson / New York Times)
- OpenAI urged the US to work with its allies, including Middle Eastern countries, in what it calls a “North American Compact for AI” to develop AI systems and compete with China. (Jackie Davalos / Bloomberg)
- Meta must face an FTC lawsuit that alleges it acquired Instagram and WhatsApp to suppress competition in social media, a judge ruled. (Jody Godoy / Reuters)
- Meta said it is cutting the price of its ad-free subscriptions for Facebook and Instagram in the EU by 40 percent to comply with regulations (Jonathan Vanian / CNBC)
- Meta was fined almost €800 million for imposing unfair trading conditions on other providers by tying its Facebook Marketplace services with the social network. Europe really has it out for Meta these days. (Javier Espinoza / Financial Times)
- The Consumer Financial Protection Bureau is reportedly seeking to place Google under federal supervision that would subject the search giant to regular inspections. (Tony Romm / Washington Post)
- Amazon executives were reportedly called in for a meeting with a key House committee to answer questions over the shopping partnership with TikTok that it maintains despite a looming ban. On the other hand, how large is that ban actually looming these days? (Alexandra S. Levine / Bloomberg)
- Chinese hackers stole “private communications” of a “limited number” of government officials after accessing multiple US broadband providers, the FBI and CISA said. (Sergiu Gatlan / BleepingComputer)
- Apple is facing a $3.8 billion antitrust lawsuit from UK consumer rights group Which? for allegedly locking people into paying for iCloud at “rip-off” prices. I'm sorry but Which??? (Natasha Lomas / TechCrunch)
- Google is testing the removal of news articles from EU publishers on Search, which it says is because regulators “asked for additional data about the effect of news content in Search.” (Emma Roth / The Verge)
- Google will stop serving political ads to EU users, it said, due to uncertainties around new transparency regulations. (Jess Weatherbed / The Verge)
- The EU’s AI Act’s Code of Practice has a first draft that touches on copyright, systemic risks and transparency requirements. (Natasha Lomas / TechCrunch)
- A look at the development of the spyware market in Italy. (Suzanne Smalley / The Record)
- Australia’s new Digital Duty of Care law would require social media companies to take proactive steps to address mental health risks for users, particularly children, online. (Nicole Hegarty / Australian Broadcasting Corporation)
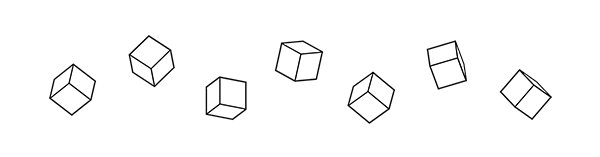
Industry
- A look at the confusion that ensued after competitors found out how quickly Musk built his supercomputer for xAI. (Anissa Gardizy / The Information)
- X reportedly appointed Mahmoud Reza Banki, former CFO of streaming platform Tubi, as its new CFO. (Alexa Corse and Becky Peterson / Wall Street Journal)
- Mark Zuckerberg dropped a cover of “Get Low” with T-Pain, dedicated to his wife. They call themselves Z-Pain. (Emma Roth / The Verge)
- Meta is reportedly planning to launch ads on Threads in January 2025. (Sylvia Varnham O’Regan and Kalley Huang / The Information)
- Threads added 15 million users this month, Instagram head Adam Mosseri said. (Jay Peters / The Verge)
- YouTube is testing a TikTok-like feed that shows endless videos when you scroll under the video that you’re currently watching. (David Pierce / The Verge)
- YouTube users can soon buy “jewels” to exchange for gifts to send eligible creators in the YouTube Partner Program. (Umar Shakir / The Verge)
- Google DeepMind released the source code and model weights for AlphaFold 3, which can model complex interactions between proteins and other molecules. (Michael Nuñez / VentureBeat)
- AI pioneer and French developer François Chollet is leaving Google after nearly a decade. (Kyle Wiggers / TechCrunch)
- Google is rolling out real-time Scam Detection for phone calls on Pixel devices, which will listen for “patterns commonly associated with scams” and send visual warnings. (Abner Li / 9to5Google)
- Google Maps users can now search for items like home goods and clothing in nearby stores. (Jess Weatherbed / The Verge)
- Amazon is reportedly making the process for disabled employees to get permission to work from home more difficult, establishing a rigorous and sometimes invasive vetting process. (Spencer Soper / Bloomberg)
- Apple released updates to Final Cut Pro for the Mac and iPad, including features like Live Drawing and an updated camera app. (John Voorhees / MacStories)
- Spotify will start paying creators and podcast hosts for videos, based on the engagement the videos receive from paid subscribers. (Alex Heath / The Verge)
- The move seems to be aimed at gaining back viewers that turn to YouTube for video podcasts. (Anne Steele / Wall Street Journal)
- A Q&A with Gustav Söderström, Spotify’s co-president, chief technology officer and chief product officer, on the company’s plans for generative AI. (Alex Kantrowitz / Big Technology)
- Parents will soon be able to request their children’s locations on Snap in the Family Center and more easily see who their child is sharing their location with. (Quentyn Kennemer / The Verge)
- AI search engine Perplexity will begin testing ads on its platform this week, the company said. (Kyle Wiggers / TechCrunch)
- Openvibe, a multi-network app, lets users post to three X alternatives – Mastodon, Bluesky and Threads – at once. (Harry McCracken / Fast Company)
- The Wall Street Journal is testing AI-generated summaries of its articles, appearing as a “Key Points” box at the top of news stories. (Jay Peters / The Verge)
- A look at how the art world is drawing inspiration from AI to explore ethical issues and its risks. (Erin Griffith / New York Times)
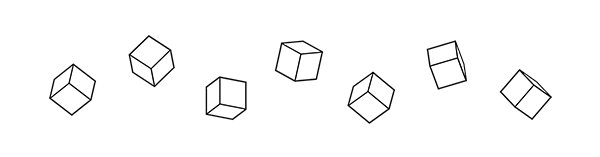
Those good posts
For more good posts every day, follow Casey’s Instagram stories.
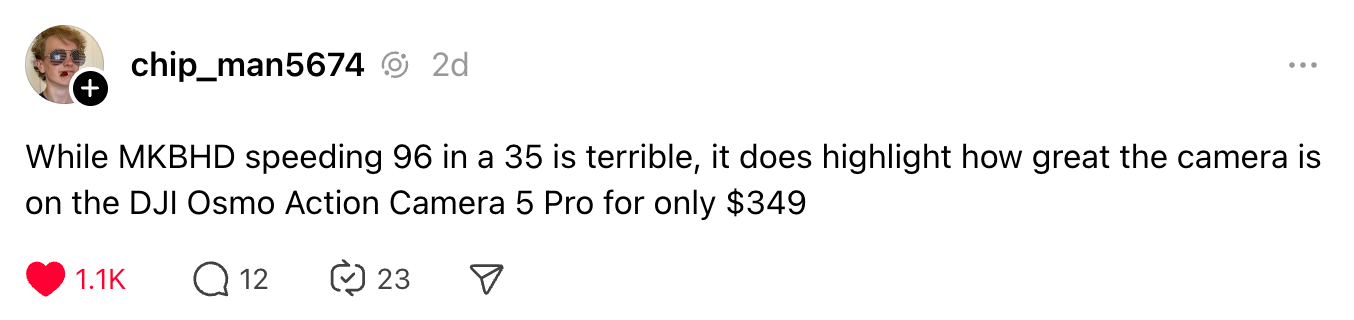
(Link)
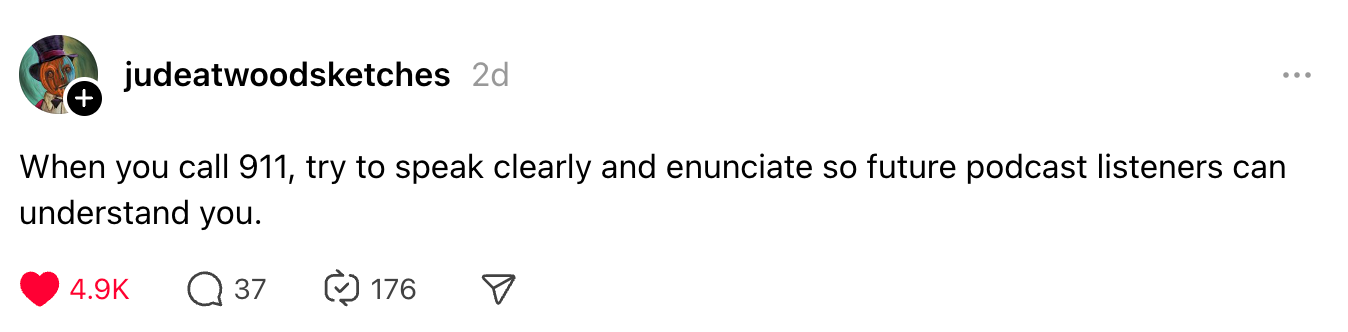
(Link)
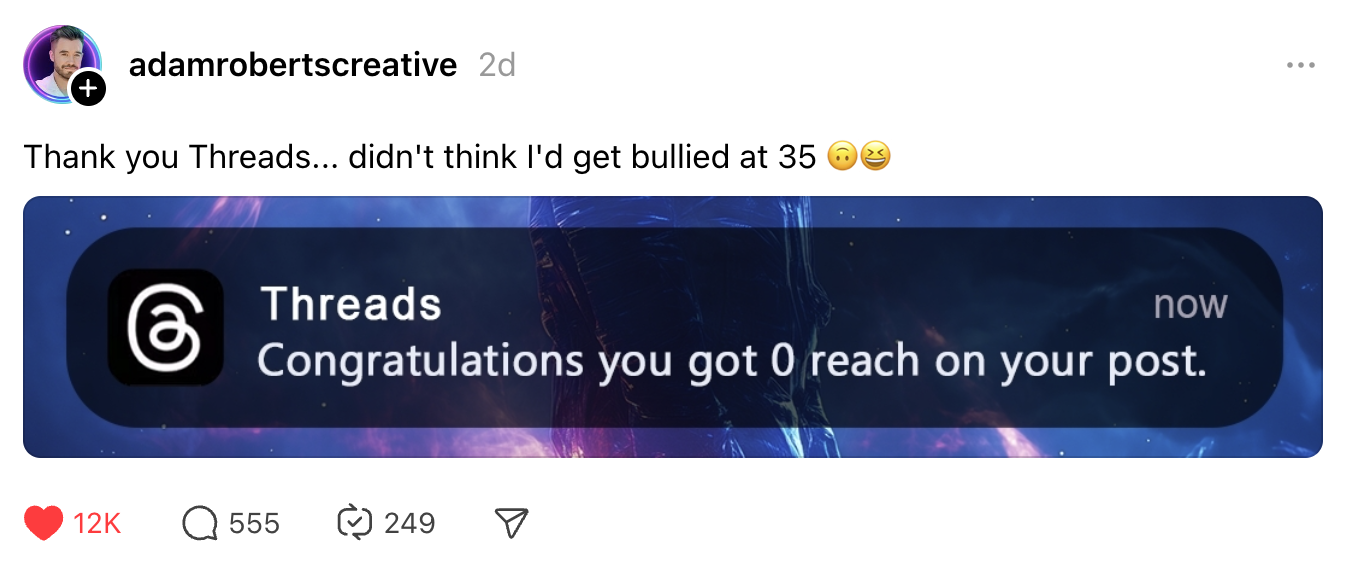
(Link)
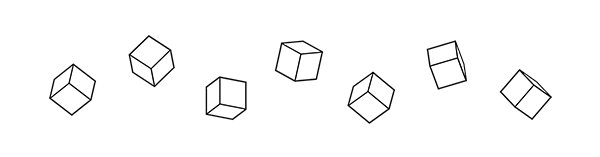
Talk to us
Send us tips, comments, questions, and scaling law workarounds: casey@platformer.news.