Three reasons we’re in an AI bubble (and four reasons we’re not)
AI critics are right about the industry’s challenges — but they risk missing the larger story
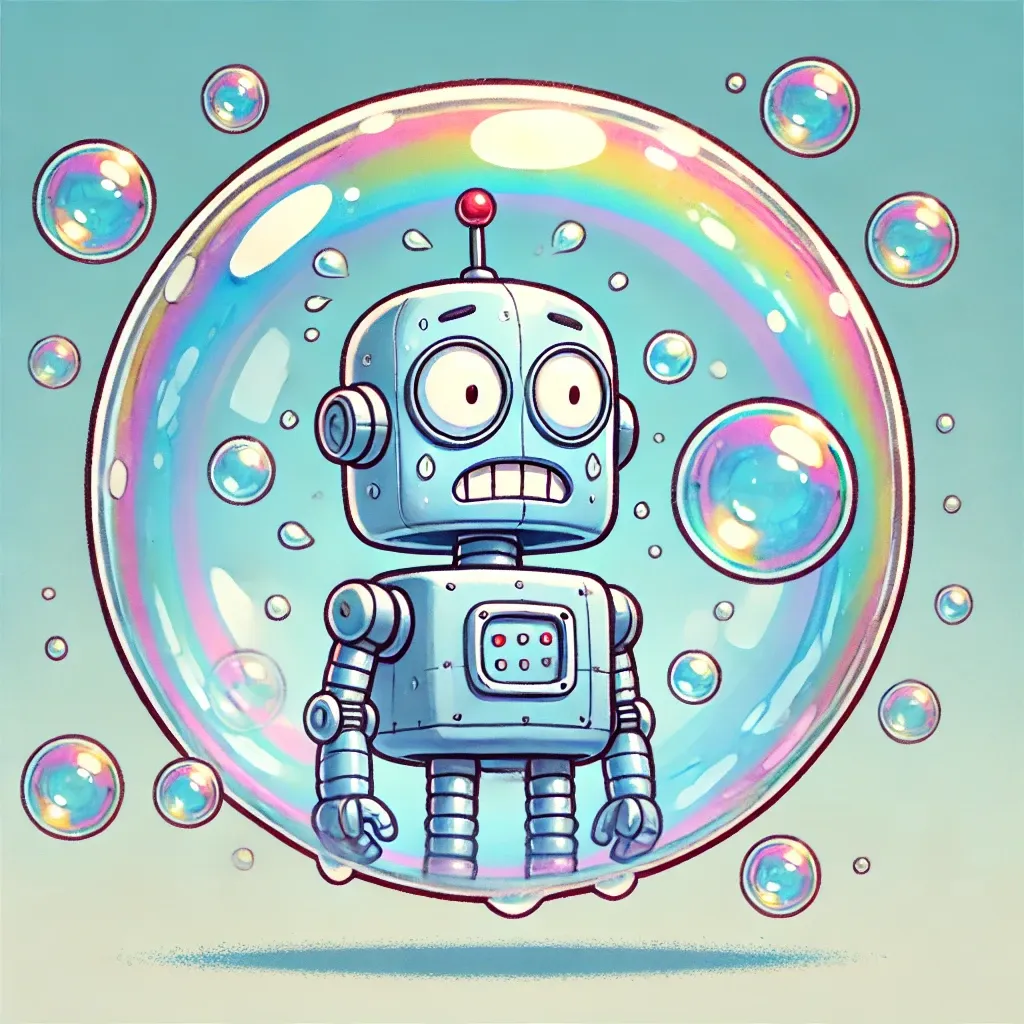
When tech stocks plunged on Monday, temporarily losing a collective $800 billion in value, a long-simmering debate about artificial intelligence boiled over. Almost two years after ChatGPT was released, tech giants are investing more than ever into generative AI and the promise of superintelligence. But with capital expenditures far outstripping AI profits and the pace of innovation seeming to slow, investors are beginning to wonder when Silicon Valley plans to recoup those expenses.
Are we living through an AI bubble? The prominent hedge fund Elliott Management raised eyebrows earlier this week when it told clients that big technology stocks, including Nvidia, are in “bubble land,” the Financial Times reported. Elliott expressed skepticism that demand for Nvidia’s powerful chips would persist, and described AI in general as “overhyped with many applications not ready for prime time.”
It’s possible that AI applications are “never going to be cost-efficient, are never going to actually work right, will take up too much energy, or will prove to be untrustworthy,” the hedge fund wrote.
Elliott has a point: many AI tools today absolutely are not “ready for prime time,” in the sense that you could trust them with your job or your life. AI needs close supervision, sometimes requiring more effort than it would take to simply have done the task yourself.
At the same time, talk to most software engineers today and they’ll tell you they already can’t imagine doing their jobs without the coding assistance that AI provides. Are they the exception to the rule — or just early in realizing the kind of productivity gains that will come to the rest of the economy over time?
Whether we’re in an AI bubble depends largely on how you define it. When I use the term, I’m talking about a situation where public company stocks and private company valuations are inflated far beyond the profits they will ever deliver, creating the conditions for a huge crash when speculators pull out and prices decline. The dot-com crash of the late 1990s is the canonical example in Silicon Valley.
Are we setting ourselves up for an AI sequel? There’s a case for and against it. Let’s start off with some reasons why the AI bubble might be real.
Companies aren’t yet earning profits on their AI investments, and it’s not clear if or when they will.
Investors like Elliott want to see Silicon Valley pull back on their AI spending this year. But tech giants are doing the opposite: Microsoft, Alphabet, Amazon and Meta all increased expenditures on AI dramatically in the first half of this year, to a collective $106 billion. Some analysts believe they will spend $1 trillion on AI over the next five years, the FT reported.
To recoup those costs, the giants will need to persuade vast numbers of consumers and businesses to buy their services. But for now enterprise spending on AI is mostly limited to small trials, and there’s little evidence so far that most businesses see a compelling reason to buy the tools that are now available. Plenty of people who tried ChatGPT once or twice never returned. As Elliott put it: “There are few real uses … [beyond] summarizing notes of meetings, generating reports and helping with computer coding.”
Moreover, AI tools have much lower profit margins than other software, thanks to their intensive computing demands and energy needs. So the cost of providing AI services scales with usage in a way that just isn’t true of (for example) Google search or Facebook.
Some of the most prominent startups are throwing in the towel.
Character.AI raised $150 million to build AI chatbots. Adept raised $415 million to build AI agents. Inflection raised a whopping $1.525 billion to build an AI chatbot named Pi.
All of these companies still exist in some form. But they no longer have their founders, who agreed to go work for tech giants in a series of non-acquisition acquisitions that have become the norm for AI upstarts this year. In each case, the non-acquirers (Google for Character, Amazon for Adept, and Microsoft for Inflection) paid investors a modest premium. But venture capitalists are surely stinging that some of the most promising companies in the space failed to deliver the 10x returns that their funds are depending on.
What gives? Regulators are increasingly skeptical about mergers and acquisitions in the tech world, closing off the most popular path for startups to exit. Meanwhile, startups are learning that even billion-dollar fundraises can’t compete with what the giants are prepared to spend to win the AI race. That makes the prospect of taking a company public especially daunting.
“If the very best outcome for extremely well-funded AI companies are deals like these, then it signals that building a standalone, potentially profitable GenAI business is too hard or impossible to pull off,” Gergely Orosz, author of the Pragmatic Engineer newsletter, wrote this week. “We can assume founders are smart people, so throwing in the towel early by selling to Big Tech is likely to be the most optimal outcome.”
The rate of innovation seems to be slowing down.
One reason why ChatGPT created a sensation is that it improved dramatically on the output of the previous version of OpenAI’s large language model. The leap from GPT-2 to GPT-3 was remarkable: a tool that previously had hardly any utility to the average person was suddenly capable of helping a student cheat all the way through elementary school.
GPT-4, and later GPT-4o, are even better: they’re faster, more efficient, and less likely to hallucinate than GPT-3. But we still use them just the same as we used ChatGPT, and they have few capabilities that their predecessor did not.
For the past several years, AI developers have been able to create much more powerful models simply by increasing the amount of data that their models are trained on. But there are signs that this approach is beginning to show diminishing returns. Until GPT-5 and other next-generation models arrive in the next year or so, it will remain an open question.
Taken together, these factors could reasonably lead a person to believe that the bubble talk is real. So what’s the case against?
Tech companies are often unprofitable for very long stretches.
Amazon didn’t turn a profit for the first nine years of its life. Uber reported its first full-year profit this year — 15 years after it was founded. The end of zero-interest rates has made it much more difficult for tech companies to operate this way. But particularly for the richest public companies, making long-term investments and ignoring investors’ complaints has long been the norm.
Big Tech CEOs are all largely in agreement that AI represents their largest opportunity in at least a generation. In their view, to pull back on spending now would risk ceding the race to their competitors — which would be even worse for their long-term profits. “In tech, when you are going through transitions like this . . . the risk of underinvesting is dramatically higher than overinvesting,” Google CEO Sundar Pichai told investors on an earnings call last month.
Startups failing is a normal part of the venture capital life cycle.
Just because a handful of once-high flyers like Character.AI went looking for the exits doesn’t mean the entire industry is washing out. Just ask the image generator Midjourney, which was expected to generate $200 million last year and has reportedly been profitable since its earliest days. Or ask OpenAI, whose ChatGPT app just had its best ever month in revenue, according to estimates from market research firm app intelligence. (That seems particularly notable given that most American children are not in school in July.)
Tech companies are still wringing plenty of innovation out of current generation models.
Whether GPT-5 and its peers can deliver a step-change in functionality from their predecessors is a real and important question. But focusing on that too much can obscure just how much innovation is left to be wrung out of the models we have today.
To get a sense of what’s still possible, keep an eye on Google DeepMind. Last month, the company used a pair of custom models to earn the equivalent of a silver medal at the International Mathematical Olympiad. (It was one point short of the gold.) Today, they showed off an amateur table tennis robot that beat every beginner who stepped up to face it. (And lost to every advanced player.) And those feats came just a couple months after DeepMind unveiled AlphaFold 3, which predicts protein structures with amazing accuracy.
There’s no telling how long it will take Google to recoup its investment in DeepMind. But are there billions to be made in robotics, medicine, and health care? To me the answer seems obviously to be yes.
Technology adoption takes a long time.
Credit to my podcast co-host, Kevin Roose, for pointing this one out to me. Microwave ovens were invented in 1947, and by 1971 were only in 1 percent of American homes. They didn’t reach 90 percent of American homes until 1997.
Other technologies proliferate more quickly. ChatGPT set a record for the fastest-growing consumer application ever. And yet it also remains true that, as with the microwave in the 1970s, most people have never tried it. That’s why I’m most interested in what natural early adopters, like software engineers, are doing with AI. The more success they have with Copilot and other AI assistance, the more others will seek out similar tools. But that’s not all going to happen this year.
So how do we square these two cases?
Here’s my view. AI seems to be creating much bigger opportunities for our biggest companies than our smallest ones. The tech giants can easily afford to plow billions into building next-generation LLMs and subsidizing AI services while they work to bring costs down and scale user bases up. And even if the direst predictions of AI skeptics came true, and no one ever found a profitable use for generative AI, the tech giants would all still have their massive profitable businesses in e-commerce, advertising, and hardware to prevent a true collapse.
On the other hand, startups have a much harder road. The natural advantages they normally have over giants, such as being small and nimble, can’t make up for the high cost of training models and offering AI-powered services. VC portfolios are sufficiently diversified that even their outsized bets on AI won’t sink most of them, just as making outsized bets on crypto didn’t sink most of them. But if AI remains on its current trajectory — and regulators don’t allow more acquisitions — I suspect VCs will be really disappointed.
But even if we are in a bubble, don’t expect that to be the last word on AI — any more than the dot-com bubble marked the end of the internet. Sometimes technologies that in the moment look too expensive or too unreliable in hindsight only look too early.
Correction: This post originally said ChatGPT had been available for almost three years. In fact, it was been available for almost two.
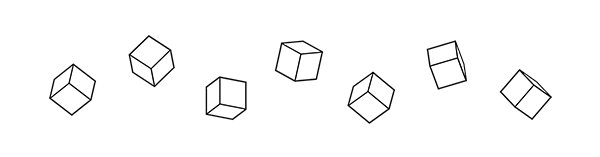
Launches
For several years now I've found Oliver Darcy's Reliable Sources newsletter at CNN to be essential reading. Today Oliver announced he's striking out on his own with a new newsletter about the media industry called Status.
It was an instant annual subscription purchase for me. If you want to support high-quality, independent media reporting, check it out.
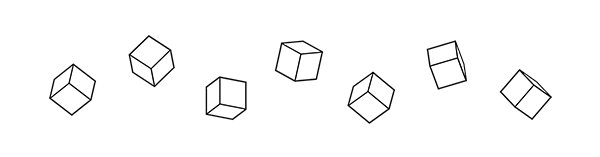
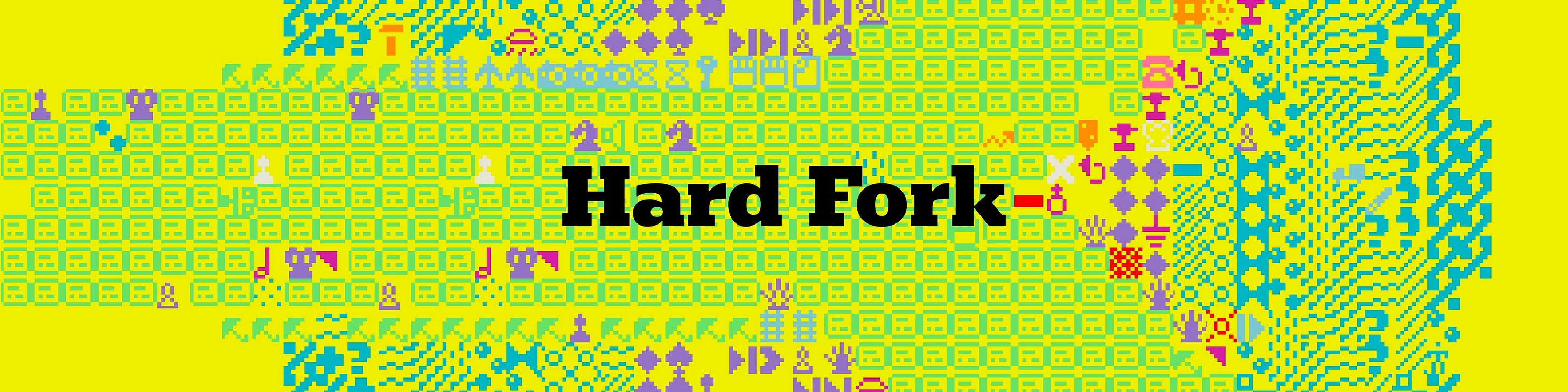
On the podcast this week: The Times' David McCabe joins us to sort through this week's ruling that Google has illegally maintained its monopoly in search. Then, Kevin and I talk through the AI bubble debate. And finally, all aboard for a new segment we call Hot Mess Express.
Apple | Spotify | Stitcher | Amazon | Google | YouTube
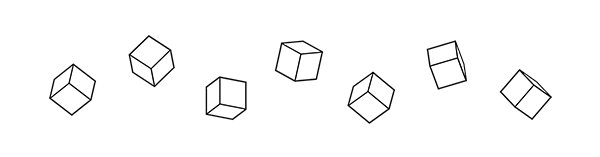
Governing
- UK authorities asked a number of social media platforms to take down posts that it deemed was harmful to national security, but is facing resistance from X. (Anna Gross, Stephanie Stacey and Hannah Murphy / Financial Times)
- Disinformation on X fueled the UK riots, this columnist writes, and exposed a regulatory blindspot in the country. (Parmy Olson / Bloomberg)
- The UK government is reportedly considering a revival of a provision in the Online Safety Act that would give authorities more enforcement power over social media content moderation. (Ellen Milligan and Mark Bergen / Bloomberg)
- Ofcom, the UK’s internet authority, published an open letter to social media platforms over concerns about the use of their tools to incite violence. (Natasha Lomas / TechCrunch)
- Elon Musk deleted a post on X that stemmed from a fake news headline of how British prime minister Keir Starmer was considering building detainment camps. But not before it got millions of views. (Josh Self / Politics.co.uk)
- Telegram, a messaging app with lax content moderation tools, saw a surge in use as extremist groups turned to the platform to mobilize after the riots. (Stephanie Stacey, Anna Gross and Hannah Murphy / Financial Times)
- The World Federation of Advertisers disbanded the Global Alliance for Responsible Media a day after X filed a lawsuit accusing it of antitrust violations. A stunning capitulation to Elon Musk, Jim Jordan, and those who are attempting to legally compel private corporations to advertise on conservative media. (Lara O'Reilly / Business Insider)
- Kamala Harris’s campaign raised $150,000 through VCs for Kamala, led by venture capital firm SV Angel’s founder and managing partner, Ron Conway. (Lauren Feiner / The Verge)
- The most pro-Trump ads are on Truth Social, according to this analysis, with Trump Media relying on the niche ad market for revenue. (Matthew Goldstein, David Yaffe-Bellany and Stuart A. Thompson / New York Times)
- California’s AI bill will harm the US’s budding ecosystem, says Fei-Fei Li, the ‘Godmother of AI,’ and doesn’t address potential harms of AI advancement like bias and deepfakes. (Fei-Fei Li / Fortune)
- A look at the privacy concerns emerging from social media age checks that often use AI to scan children’s faces. (Drew Harwell / Washington Post)
- Facebook creators who violate Community Standards for the first time will now be offered the option to take a training course, which leads to removal of the imposed warning upon completion. YouTube did something similar, and I love it. More like this, please. (Sarah Perez / TechCrunch)
- X removed all ads from its top subscription tier amid its lawsuit against an ad industry group. (Sarah Perez / TechCrunch)
- The UK’s competition authority formally launched an inquiry into Amazon’s $4 billion investment in AI startup Anthropic. (Camilla Hodgson / Financial Times)
- Apple revised its rules for the App Store in the EU to ease back on restrictions for developers and added a new commission fee structure to comply with the Digital Markets Act. (Natasha Lomas / TechCrunch)
- Spotify and Epic Games say the revisions are still unacceptable and include “junk fees” that are illegal. (Sarah Perez / TechCrunch)
- Grok will not be trained on X posts by European users for the time being, following concerns from Ireland’s Data Protection Commission. (Natasha Lomas / TechCrunch)
- Roblox was banned in Türkiye following reports of inappropriate sexual content on the platform. (Tükiye Today)
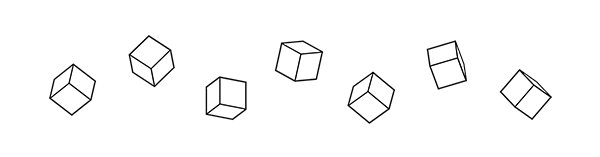
Industry
- Intel reportedly had the option to buy a stake in OpenAI seven years ago, but declined after then-CEO Bob Swan decided that it was unlikely that generative AI models would make it to market in the near future. (Max A. Cherney / Reuters)
- OpenAI is reportedly leading a $60 million funding round for hardware startup Opal. Why?? (Stephanie Palazzolo and Kate Clark / The Information)
- OpenAI added Zico Kolter, a machine learning professor focused on AI safety at Carnegie Mellon, to its board of directors. (Kyle Wiggers / TechCrunch)
- Free users of ChatGPT can now generate up to two images per day with DALL-E 3. (Jay Peters / The Verge)
- TikTok is adding an in-app hub, called “TikTok Spotlight,” for movies and TV shows. They'll offer ticketing details and other information on users' videos about the movies and shows in question. (Emma Roth / The Verge)
- Views will be the primary metric for measuring content on Instagram, Adam Mosseri said, so that creators can track how well content is doing across different formats. (Jay Peters / The Verge)
- Meta is reportedly in talks to license its Horizon VR software to Indian tech company Jio. (Sylvia Varnham O’Regan, Wayne Ma and Amir Efrati / The Information)
- Meta is also reportedly closing down Ready at Dawn Studios, the VR development studio behind Echo VR and Lone Echo games. (Nicholas Sutrich / Android Central)
- Google and Meta reportedly struck a secret ad deal that involved targeting YouTube users ages 13 to 17 with Instagram ads. (Stephen Morris, Hannah Murphy and Hannah McCarthy / Financial Times)
- Instagram is adding the ability for users to add up to 20 photos or videos to their feed carousels, embracing the “photo dump” phenomenon. (Aisha Malik / TechCrunch)
- YouTubers that make car content are quitting the channels that they helped popularize following acquisitions by companies backed by private equity firms. (Tim Stevens / The Verge)
- Reddit beat analyst expectations in its second-quarter results, reporting a boost in revenue from data-licensing along with a comeback in advertising revenue. (Jonathan Vanian / CNBC)
- Reddit is testing AI-generated summaries at the top of its search results. It may also test paid subreddits. (Lauren Forristal / TechCrunch)
- The role of AI in Hollywood has become a polarizing issue between entertainers and studios as studios increasingly adopt the tech in the production pipeline. (Winston Cho / Hollywood Reporter)
- Bizarre AI-generated cat videos with storylines – sometimes grotesque and dark ones – are becoming a content niche online. (Taylor Lorenz / Washington Post)
- WordPress.com owner Automattic launched an AI tool that aimed at helping bloggers write more succinctly. (Paul Sawers / TechCrunch)
- Humane, maker of the AI Pin, is reportedly seeing greater returns than sales in recent months. (Kylie Robison / The Verge)
- Bumble shares fell as much as 32 percent after it slashed its growth outlook by at least 7 percent. (Evan Gorelick / Bloomberg)
- A look at the changes Mozilla is making to the Firefox browser and its alienation of some loyal users over privacy concerns. (Jared Newman / Fast Company)
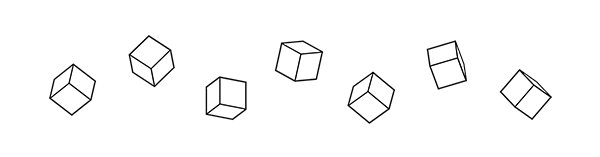
Those good posts
For more good posts every day, follow Casey’s Instagram stories.
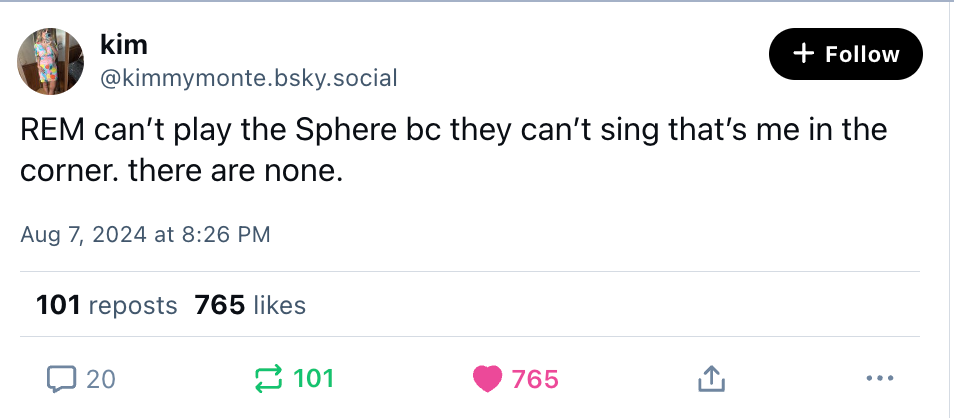
(Link)
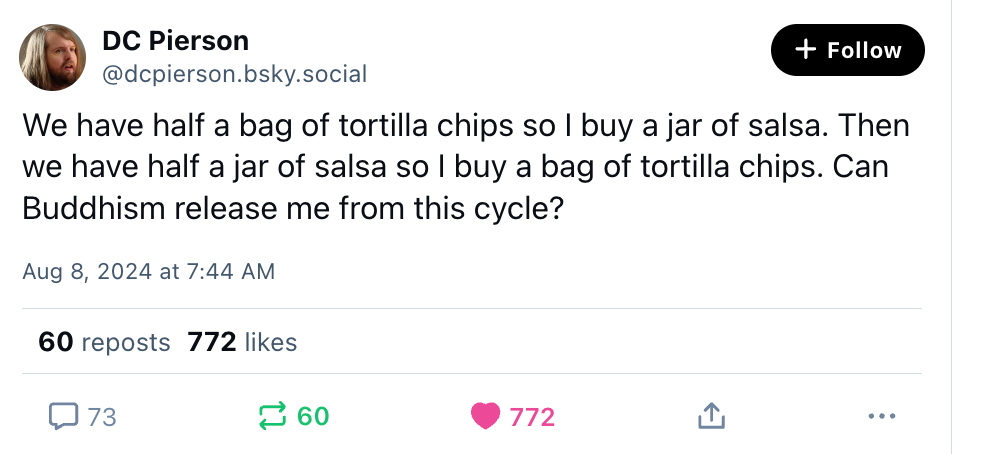
(Link)
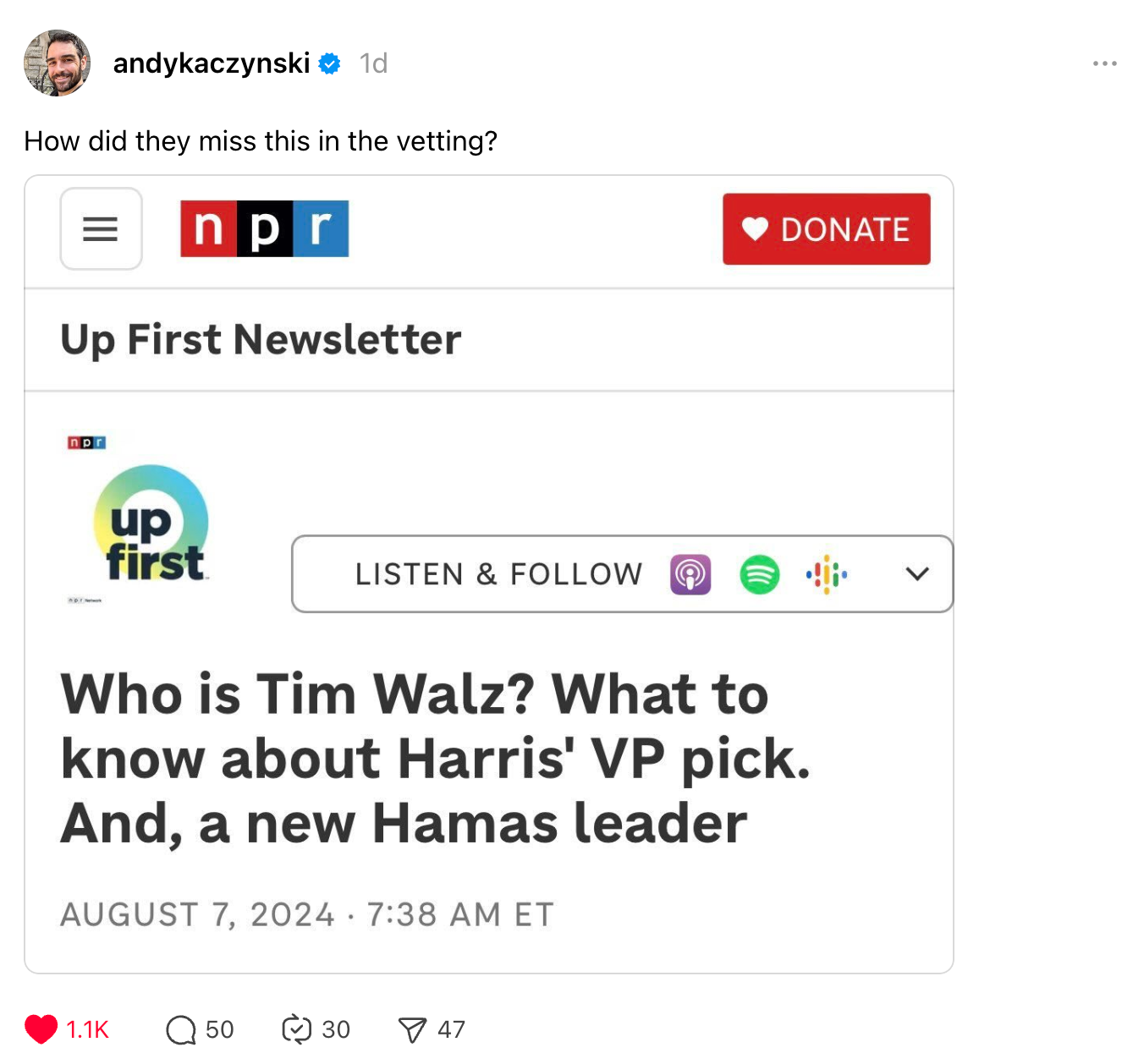
(Link)
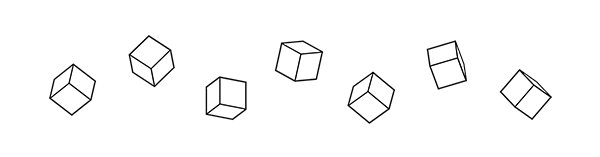
Talk to us
Send us tips, comments, questions, and AI bubble opinions: casey@platformer.news.